Machine learning is a branch of artificial intelligence technology that involves developing algorithms and models that enable computers to learn from data without being explicitly programmed. In other words, machine learning is teaching machines to recognize patterns and make predictions based on data rather than relying on explicit instructions.
Machine learning has become increasingly important in recent years due to the explosion of available data and the need to automate and improve decision-making processes in various industries. With the ability to process vast amounts of data quickly and accurately, machine learning has the potential to revolutionize everything from healthcare and finance to transportation and entertainment.
There are three main types of machine learning: supervised learning, unsupervised learning, and reinforcement learning. In supervised learning, the machines trained on labelled data, where the correct answer is provided for each example. In unsupervised learning, the device is trained on unlabelled data and must find patterns and structures independently. Reinforcement learning involves teaching a machine to take actions in an environment to maximize a reward signal.
In this guide, we will explore the key concepts and techniques of machine learning, including data preprocessing, model selection, and evaluation metrics. We will also discuss some of the most common machine learning algorithms, their applications, and potential ethical considerations.
-
Key Concepts
To understand the basics of machine learning, there are several key concepts that you should be familiar with:
- Data: The foundation of machine learning is data. This includes both the input data (known as features) and the output data (known as labels or targets). The quality and quantity of the data will directly impact the accuracy and effectiveness of the machine-learning algorithm.
- Features: Features are the individual attributes or characteristics of the input data that the machine learning algorithm uses to make predictions. For example, in a dataset of housing prices, the features might include the number of bedrooms, the size of the lot, and the age of the house.
- Models: A model is a mathematical representation of the relationship between the data’s features and labels. Machine learning algorithms use these models to make predictions based on new, unseen data.
- Algorithms: Algorithms are the specific mathematical and statistical techniques used to train the machine learning model. Different algorithms are better suited to different types of problems and data.
- Training: Training a machine learning algorithm involves feeding it data and adjusting the model’s parameters to minimize the difference between the predicted output and the actual output.
- Testing: Once a model has been trained, it must be evaluated on new, unseen data to assess its accuracy and generalizability.
- Prediction: The ultimate goal of a machine learning algorithm is to use the trained model to make predictions on new data, allowing for automated decision-making or improved insights.
Understanding these key concepts is essential to effectively working with machine learning algorithms and interpreting their results. The following sections will explore these concepts in more detail, starting with data pre-processing.
-
Data Pre-processing
Data pre-processing is a critical step in machine learning, as it helps to ensure that the data is in a suitable format for training and testing machine learning algorithms. This involves several tasks:
- Cleaning data: Data cleaning involves identifying and correcting errors or inconsistencies in the data, such as missing values, outliers, and incorrect data types.
- Handling missing data: Missing data can be a common problem in datasets. Several strategies for managing missing data include removing rows or columns with missing values, imputing values based on the mean or median, or using more advanced techniques such as regression or machine learning.
- Feature scaling: Feature scaling involves transforming the data so each feature is on a similar scale. This can help improve the performance of some machine learning algorithms, particularly those sensitive to the input data’s scale.
- Feature selection: Feature selection involves identifying the most important features in the data and removing those that are redundant or irrelevant to the problem. This can help to simplify the model and improve its accuracy.
-
Supervised Learning
Supervised learning is a type of machine learning where the algorithm learns from labelled data to make predictions or classifications on new, unseen data. In other words, the algorithm is trained on a set of input-output pairs, where the output is known and provided in the training data, and then it learns to predict the outcome for new input data.
There are two main types of supervised learning:
- Regression: In regression, the goal is to predict a continuous output variable. This might include predicting housing prices based on features such as the number of bedrooms, the size of the lot, and the age of the house or indicating the amount of rainfall based on temperature and humidity data.
- Classification: In classification, the goal is to predict a categorical output variable. This might include classifying emails as spam or not spam or classifying images of animals into different categories.
Some standard algorithms used in supervised learning include:
- Linear regression: Linear regression is a simple algorithm that models the relationship between the input and output variables as a straight line. It is commonly used for regression problems.
- Logistic regression: Logistic regression is a classification algorithm that models the probability of each class as a logistic function of the input variables.
- Decision trees: Decision trees are a popular algorithm for both regression and classification. They divide the input space into regions based on the values of the input variables and assign a prediction based on the majority class or the average value in each area.
- Random forests: Random forests are an ensemble method that combines multiple decision trees to improve their accuracy and reduce overfitting.
- Support vector machines: Support vector machines are robust algorithms for classification that attempt to find a hyperplane that separates the classes in the input space.
-
Unsupervised Learning
Unsupervised learning is a type of machine learning where the algorithm learns from unlabelled data to discover hidden patterns or structures in the data. In other words, the algorithm is not provided with the output variable. Instead, it seeks to find the underlying structure of the data by grouping or clustering similar data points.
There are two main types of unsupervised learning:
- Clustering: The goal of clustering is to group similar data points together based on their features or attributes. This might include grouping customers with similar purchasing habits or images with similar visual elements.
- Dimensionality reduction: In dimensionality reduction, the goal is to reduce the number of features in the data while retaining as much information as possible. This might include compressing high-dimensional data into a lower-dimensional space or identifying the most critical elements in the data.
Some standard algorithms used in unsupervised learning include:
- K-means clustering: K-means clustering is a simple and popular algorithm for clustering. It partitions the data into k clusters based on the distance between each data point and the centroids of the groups.
- Hierarchical clustering: Hierarchical clustering is a clustering algorithm that builds a hierarchy of clusters by iteratively merging or splitting sets based on the similarity of their data points.
- Principal component analysis (PCA): PCA is a dimensionality reduction algorithm that identifies the essential features in the data by finding the directions of maximum variance.
- t-SNE: t-SNE is a dimensionality reduction algorithm that is particularly effective for visualizing high-dimensional data in a lower-dimensional space.
-
Evaluation Metrics
Evaluation metrics are used to measure the performance of a machine learning algorithm on a given dataset. The choice of evaluation metric depends on the problem being solved and the goals of the machine learning project.
Here are some common evaluation metrics for both classification and regression problems:
Classification Metrics:
- Accuracy: The proportion of correct predictions out of all projections.
- Precision: The proportion of accurate positive predictions out of all optimistic predictions.
- Recall The ratio of true positive predictions out of all actual positives in the dataset.
- F1 score: A harmonic mean of precision and recall that gives equal weight to both measures.
- The area under the ROC curve (AUC-ROC): A metric that measures the performance of a binary classifier at different thresholds by plotting the true positive rate against the false positive rate.
Regression Metrics:
- Mean Squared Error (MSE): The average of the squared differences between predicted and actual values.
- Root Mean Squared Error (RMSE): The square root of the MSE.
- Mean Absolute Error (MAE): The average absolute differences between predicted and actual values.
- R-squared (R2): A metric that measures the proportion of variance in the target variable that the model explains.
It is essential to choose the right evaluation metric for the task at hand, as different metrics can give additional insights into the model’s performance. For example, in a medical diagnosis task, the recall may be more important than precision, as it is more important to avoid false negatives (i.e., missing a diagnosis) than false positives (i.e., diagnosing a healthy patient as sick). Similarly, in a regression problem where the target variable has a skewed distribution, MAE may be a more appropriate metric than MSE, as it is less sensitive to outliers.
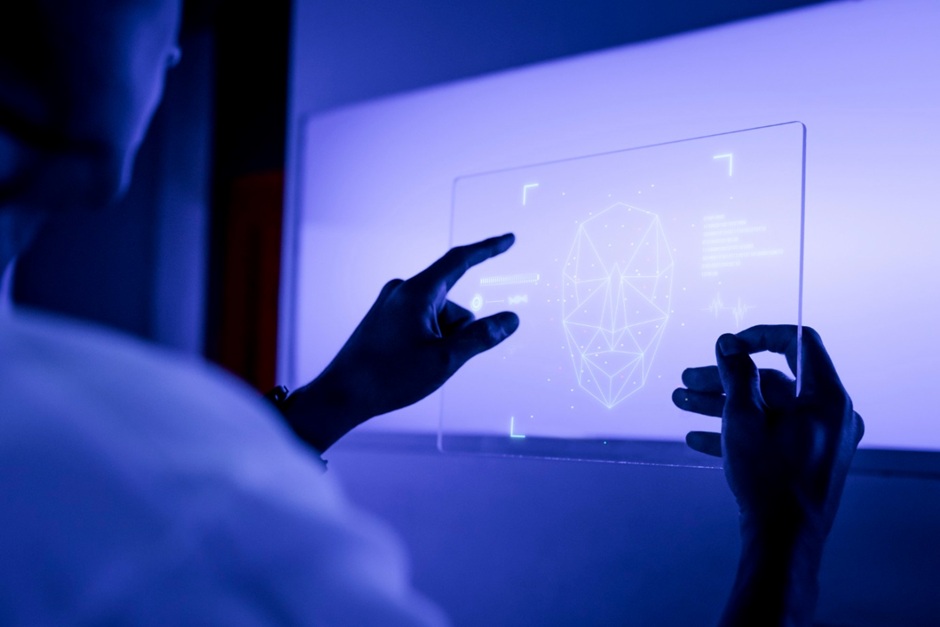
-
Model Selection and Hyperparameter Tuning
Model selection and hyperparameter tuning are essential steps in the machine-learning pipeline to improve the performance of a model.
Model Selection
A Model selection involves choosing the best algorithm for a given problem. Some standard model selection techniques include:
- Cross-validation: Cross-validation involves splitting the data into training and validation sets multiple times and evaluating the model’s performance on each split. This helps to reduce overfitting and give a more accurate estimate of the model’s performance.
- Grid search: Grid search involves exhaustively searching over a range of hyperparameters for each algorithm and selecting the combination that performs best on the validation set.
- Random Search: Random search involves randomly sampling hyperparameters from a predefined range and evaluating the performance of each combination on the validation set.
Hyperparameter Tuning
Hyperparameters are parameters that are not learned during training but are set before training. Examples of hyperparameters include the learning rate, number of hidden layers, and regularization strength. Hyperparameter tuning involves selecting the best hyperparameters for a given algorithm. Some standard hyperparameter tuning techniques include:
- Grid search: As mentioned above, grid search involves exhaustively searching over a range of hyperparameters for each algorithm and selecting the best combination on the validation set.
- Random Search: As mentioned above, random search involves randomly sampling hyperparameters from a predefined range and evaluating the performance of each combination on the validation set.
- Bayesian optimization: Bayesian optimization is a more sophisticated technique that uses prior knowledge to guide the search for the best hyperparameters. It involves building a probabilistic model of the objective function and using it to suggest hyper parameters likely to improve the model’s performance.
7. Common Machine Learning Algorithms
Many different machine learning algorithms can be used for various types of problems. Here are some common types of machine learning algorithms:
Supervised Learning Algorithms:
- Linear Regression: A linear regression model models the relationship between a dependent variable and one or more independent variables by fitting a linear equation to the data.
- Logistic Regression: A logistic regression model is used to model the probability of a binary or categorical outcome based on one or more independent variables.
- Decision Trees: A decision tree model is a tree-like model that splits the data into smaller subsets based on the values of the independent variables.
- Random Forest: A random forest model is an ensemble of decision trees that uses bagging and random feature selection to reduce overfitting.
- Support Vector Machines (SVM): A linear or nonlinear model finds the optimal hyperplane or boundary between classes.
- Naive Bayes: A Naive Bayes model is a probabilistic model that calculates each class’s probability based on the independent variables’ values.
Unsupervised Learning Algorithms:
- K-Means Clustering: A K-Means clustering model is used to group similar data points into clusters based on their distance.
- Hierarchical Clustering: A hierarchical clustering model is used to group similar data points into clusters based on their proximity.
- Principal Component Analysis (PCA): A PCA model reduces the dimensionality of a dataset by projecting it onto a lower-dimensional space while preserving the essential features.
- Association Rule Mining: Association rule mining is a technique used to find patterns or associations between variables in a dataset.
Deep Learning Algorithms:
- Convolutional Neural Networks (CNNs): A CNN model is a type of neural network used for image classification, object detection, and other computer vision tasks.
- Recurrent Neural Networks (RNNs): An RNN model is a type of neural network that is used for sequential data analysis, such as language translation, speech recognition, and time-series analysis.
- Generative Adversarial Networks (GANs): A GAN model is a type of neural network that is used for productive tasks, such as image generation, text generation, and video generation.
-
Applications of Machine Learning
Machine learning has a wide range of applications across various industries. Here are some examples of how machine learning is being used:
Image and Object Recognition:
Machine learning is used for image and object recognition tasks such as:
- Facial Recognition: Facial recognition technology is used for security and authentication purposes, as well as for social media and entertainment applications.
- Object Detection: Object detection algorithms are used for detecting objects in images or videos and are used in fields such as autonomous driving, robotics, and surveillance.
- Image Classification: Image classification algorithms are used for categorizing images based on their content and are used in fields such as medicine, agriculture, and advertising.
Natural Language Processing:
Machine learning is used for natural language processing tasks such as:
- Language Translation: Machine translation algorithms are used for translating text from one language to another in fields such as travel, commerce, and education.
- Sentiment Analysis: Sentiment analysis algorithms are used for analyzing text sentiment and in fields such as social media, customer service, and market research.
- Speech Recognition: Speech recognition algorithms are used to convert spoken language into text and in fields such as personal assistants, voice-enabled devices, and call centres.
Predictive Analytics:
Machine learning is used for predictive analytics tasks such as:
- Fraud Detection: Machine learning algorithms are used for detecting fraudulent activities and are used in fields such as finance, insurance, and e-commerce.
- Recommendation Systems: Recommendation systems are used for recommending products, services, or content to users and are used in fields such as e-commerce, entertainment, and social media.
- Demand Forecasting: Machine learning algorithms are used to predict demand for products or services in fields such as retail, transportation, and energy.
9. Ethics in Machine Learning
As machine learning algorithms become more advanced and widespread, it is essential to consider the ethical implications of their use. Here are some of the critical moral issues related to machine learning:
Bias and Discrimination:
Machine learning algorithms are only as unbiased as the data they are trained on. If the training data is biased or discriminatory, the algorithm will learn and perpetuate those biases. This can lead to discrimination against certain groups of people, such as minorities or women, in fields such as hiring, lending, and criminal justice.
Privacy:
Machine learning algorithms often require access to large amounts of personal data, such as medical records, financial information, and social media activity. It is important to ensure that this data is collected, stored, and used in a way that respects individual privacy rights and complies with relevant laws and regulations.
Transparency:
Machine learning algorithms can be opaque and difficult to understand, even for those who create them. It is essential to ensure that algorithms are transparent and explainable, so their decisions can be understood and challenged if necessary.
Accountability:
Machine learning algorithms can make decisions that have real-world consequences, such as denying a loan application or predicting a criminal risk score. It is essential to ensure accountability for these decisions and that they can be audited and reviewed.
Safety and Security:
Machine learning algorithms can be vulnerable to attacks, such as adversarial attacks, where an attacker intentionally manipulates the input data to cause the algorithm to make an incorrect decision. It is essential to ensure that algorithms are designed to be robust and secure, especially in critical applications such as autonomous vehicles and medical diagnosis.
Addressing these ethical issues requires a combination of technical solutions, such as algorithmic fairness and transparency, and legal and regulatory frameworks to protect individual rights and hold organizations accountable. It is essential for machine learning practitioners to be aware of these ethical considerations and to strive to create algorithms that are fair, transparent, and respectful of individual privacy and rights.
Conclusion
In conclusion, machine learning is a powerful tool that has the potential to revolutionize many industries and create new opportunities for innovation and growth. However, it is essential to approach machine learning with caution and to consider the ethical implications of its use. Key concepts such as data pre-processing, supervised and unsupervised learning, evaluation metrics, model selection, and hyperparameter tuning are all essential to understand when working with machine learning algorithms. Additionally, understanding standard machine learning algorithms and their applications can help identify the best approach to solve a particular problem. As machine learning continues to evolve, practitioners must prioritize transparency, fairness, privacy, and accountability to ensure that machine learning benefits society.
Author Bio
William Shakes, currently working with Averickmedia, is a content marketing expert with over seven years of experience crafting compelling articles and research reports that engage and educate audiences. With a creative mind and a passion for words, William Shakes has helped countless brands connect with their target audience through high-quality, relevant content. In addition to their exceptional writing skills, William Shakes is also a skilled strategist who can create and execute content marketing plans that drive measurable results for their clients. When not creating content, William Shakes can be found reading up on the latest industry trends or experimenting with new marketing tools and techniques.